Discovering new drugs is hard and takes a lot of time, money, and effort. Many times, the attempts to find new medicines don’t work out. But now, technology like artificial intelligence is improving and the role of AI in drug discovery can help make the drug discovery process faster and better. It has the potential to make things easier at different stages of finding new drugs, making the whole process more efficient and successful.
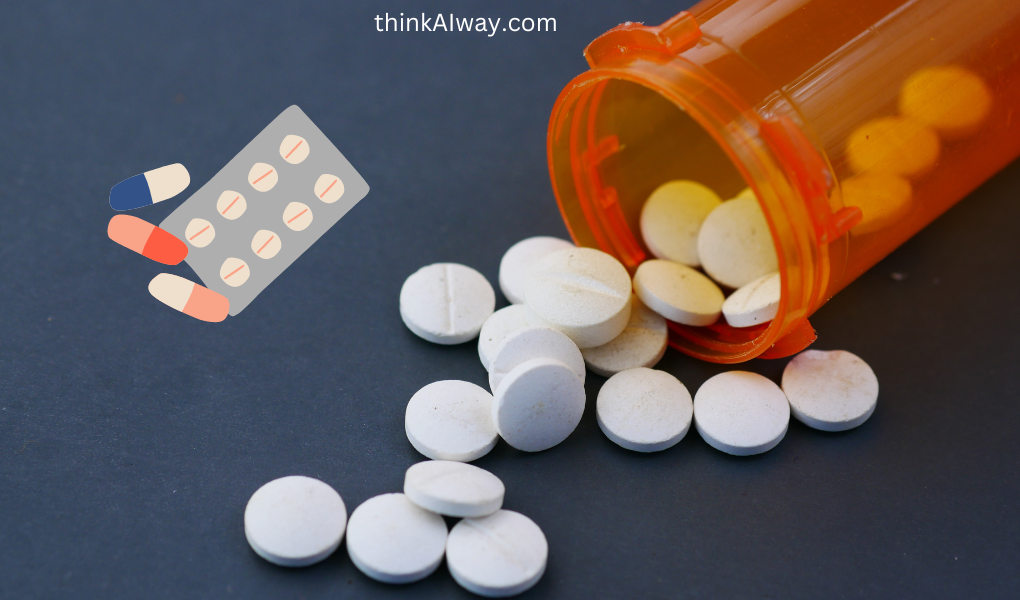
Scientists find tiny parts in our bodies, like proteins, that are important when sick. These tiny parts, such as proteins, are like the culprits causing the trouble in a disease. So, scientists try to target these trouble-causing parts with medicines to make us better. This process is crucial in drug discovery, as these parts are what we call “potential targets” for creating new drugs to treat illnesses.
Scientists are like detectives in the intricate realm of drug discovery trying to find substances that can help treat diseases. They look for chemicals or tiny particles that can work with a specific target in our bodies related to a disease. These initial findings can come from different places, like things found in nature or man-made substances created in a lab. It’s a bit like finding puzzle pieces that might fit together to create a solution for a health problem.
After finding potential substances that could be used as medicine (hits), scientists engaged in drug discovery work to make them better. They improve these hits to make them more effective, selective (targeting the right thing), and safe for use. The goal is to create a top-performing compound that has the best qualities needed for a successful drug.
Once scientists find a promising potential medicine for drug discovery, they put it through thorough testing in the lab and on animals. This is done to make sure the medicine is safe, works well, and doesn’t cause harmful effects. By doing these tests, researchers learn how the medicine behaves inside a living body, helping them figure out if it can be a successful treatment for people.
If a medicine shows promise in the early tests done in labs and on animals (preclinical testing), it moves on to the next big step – clinical trials, a crucial phase in the drug discovery process. Clinical trials are like experiments involving real people. They happen in different phases to make sure the medicine is safe, figure out the right amount to use, check how well it works, and see if there are any side effects. This helps scientists understand how the medicine behaves in a larger group of people before it can be used widely.
If a new medicine successfully finishes all the required tests and trials with positive results, the people who made it send an application called a “New Drug Application” to the government regulators. If the regulators agree that the medicine is safe and works well, they give their approval. After getting this approval, the medicine can be sold to the public, and doctors can prescribe it to patients.
Importance of integrating AI in pharmaceutical research
Using AI in pharmaceutical research is important because it can handle big sets of information, speed up finding new medicines, make it easier to identify targets, help personalize treatments, make clinical trials work better, and cut down on costs. These improvements make the process of developing drugs faster and more successful, leading to the discovery of better treatments for different illnesses. Here’s a detailed explanation of the importance of integrating AI in pharmaceutical research.
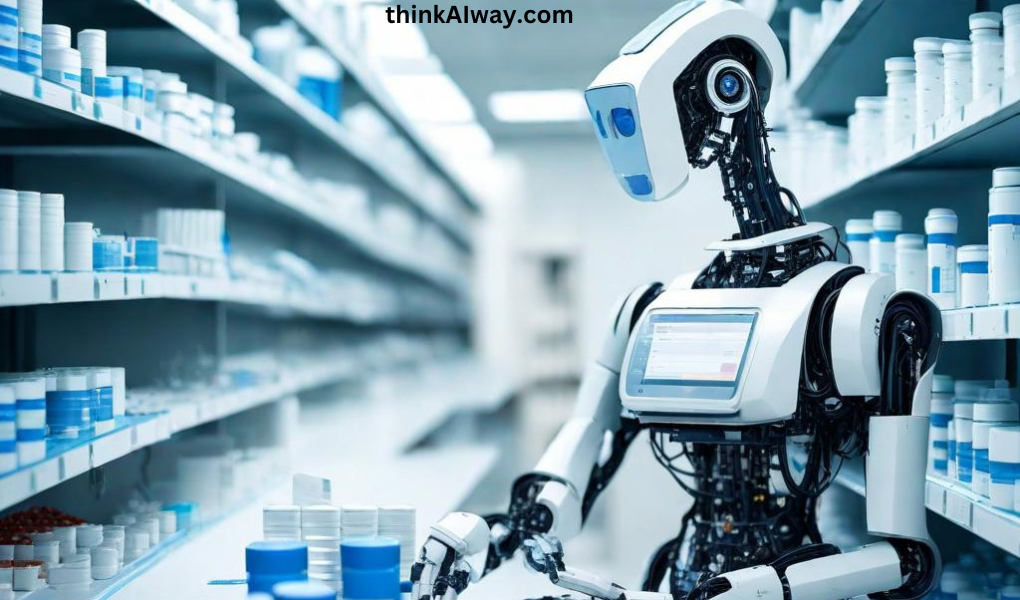
Handling Large Datasets: In drug discovery, scientists collect a lot of information from different places like genes, proteins, and tests on people. Artificial Intelligence or AI in drug discovery is good at handling all this information. It can quickly look through a huge amount of data, find important patterns, and help scientists discover important things that might be hard to find using regular methods. So, AI makes it easier for researchers to learn valuable things from all the data they collect in drug discovery studies.
Accelerating Drug Discovery: Finding new medicines through the traditional method takes a lot of time because scientists have to test many different chemicals to see which ones could be potential drugs. However, with the help of AI (Artificial Intelligence), special computer programs can guess and pick out the chemicals that are more likely to work. This makes the first step of finding possible drugs much faster and more efficient.
Enhancing Target Identification: Imagine AI as a smart helper for scientists. It can look at lots of biological information to find and check possible targets for new medicines. By studying the tiny details of how diseases work at the molecular and genetic level, scientists can use AI to pick out the most promising targets. This helps them concentrate on the things that are more likely to lead to successful new medicines.
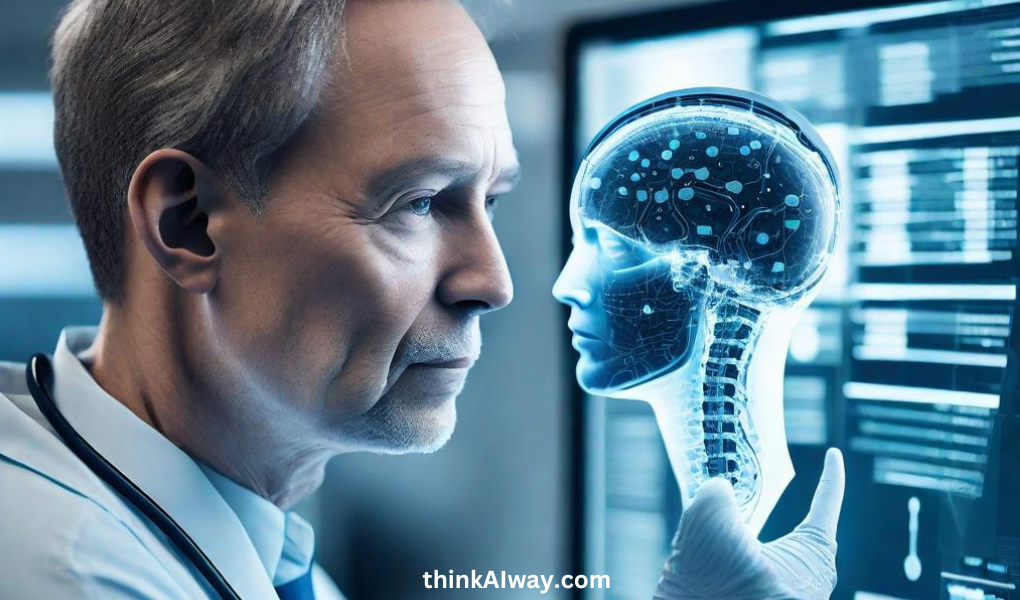
Facilitating Personalized Medicine: In simple terms, Artificial Intelligence (AI) helps create personalized treatments by looking at each patient’s information. By considering a person’s unique genetic makeup or characteristics, AI can design treatments that work better for that individual. This approach makes the treatment more effective while reducing the chances of side effects. So, it’s like getting a treatment plan that’s customized just for you, making it more efficient and safer.
Optimizing Clinical Trials: AI makes drug discovery and clinical trials smarter by selecting the right participants, determining the correct dosage, anticipating potential issues, and, in the end, improving the likelihood that the trial will be successful. Here it is explained in detail how AI significantly contributes to enhancing the efficiency and effectiveness of drug discovery processes and clinical trials.
- Finding the Right People: AI helps figure out which people with a certain condition should be part of a clinical trial. This way, the trial includes individuals who are more likely to benefit from the treatment being tested.
- Getting the Dosage Right: AI helps determine the right amount of medicine (dosage) that should be given to each person in the trial. This ensures that everyone gets the correct amount for the treatment to be effective.
- Avoiding Problems: AI can predict possible side effects or issues that might happen during the trial. This helps researchers plan to avoid problems and make the trial safer.
- Increasing Success: By doing these things, AI increases the chances that the clinical trial will succeed. It makes the whole process more efficient and helps researchers find out if a new treatment works or not.
Cost Reduction: The way we usually create new medicines is expensive and takes a long time. A big reason for this is that many experiments don’t succeed, and it takes a while to figure out which ones will work.
Now, with the help of Artificial Intelligence (AI), we can make this process more straightforward. AI helps us organize and plan better, so we don’t waste as much money on experiments that might not succeed. This means we can do research more efficiently, saving time and resources. In short, AI makes the process of creating new medicines faster and more affordable.
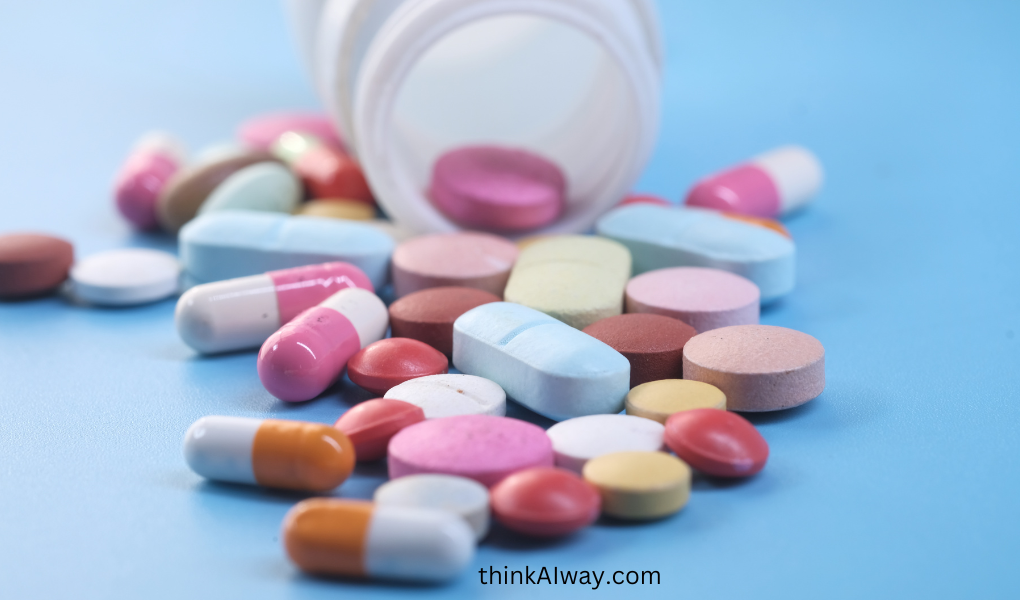
Improved Efficiency and Success Rates: AI in drug discovery plays a major role and is like a helpful assistant for scientists. It guides them to make better decisions, uses their resources wisely, and increases the chances of discovering new medicines that can help people. here it is explained in detail.
- Solving Problems: AI helps with the tricky parts of drug discovery, making it work more smoothly.
- Working Smarter: Scientists using AI in drug discovery can be smarter about how they decide what to study. It helps them choose the right things to focus on.
- Saving Resources: AI helps scientists use their time and money wisely. By making better choices, they don’t waste resources on things that might not work.
- Finding Good Medicines: With AI, there’s a better chance of finding medicines that work. It helps scientists pick the most promising candidates for further study.
Roles of AI in Drug Discovery
AI in drug discovery has a major role we will understand after reading these points. In the past, finding new medicines was a complex and slow process. Scientists had to go through a lot of tests to find the right substances in a collection that could do what they wanted, like killing bacteria for an antibiotic.
However, the collections of substances they used were so big and complicated that it was hard for individual scientists or labs to go through them all. That’s where AI and machine learning (ML) come in to help. There are some ways AI and ML help find new medicines.
Predictive Modeling: In drug discovery, scientists use these smart models to guess how well certain chemicals might work. The models use information from past experiments to predict which chemicals have the best chance of doing what they want them to do. This helps scientists decide which chemicals to focus on, making their work faster and more efficient. They can put more effort into the chemicals that are likely to be the most helpful.
Pattern Recognition: When it comes to biological data, like how genes behave or how proteins interact, machine learning helps scientists see patterns. These patterns help us understand how diseases work and find possible targets for new medicines. Imagine you have a lot of data about how different genes in our bodies act or how proteins interact. It’s like having a big puzzle. Machine learning is like a super-smart friend who can quickly figure out the patterns in a puzzle. By doing this, it helps scientists learn more about how diseases happen and find ways to create new medicines to treat them.
Quantitative Structure-Activity Relationship (QSAR) Models: Understanding how a molecule behaves helps scientists figure out if it can be a good medicine. Scientists often use these models in drug discovery. These models can tell scientists how changing the structure of a chemical might affect what it does in the body. This helps them design medicines that work better.
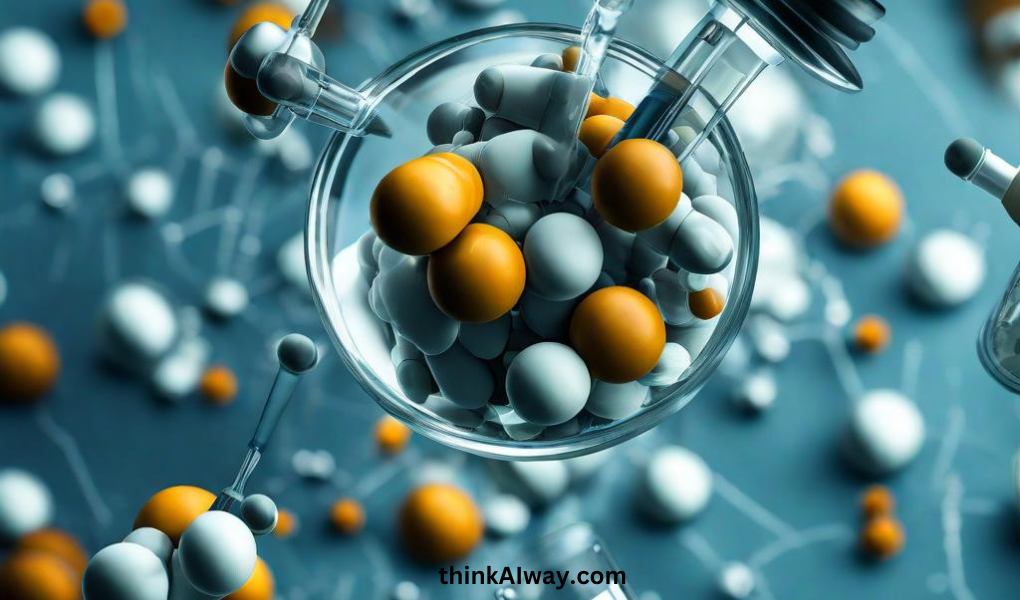
Virtual Screening: ML algorithms have a special skill, they can examine the shapes and structures of molecules and predict which ones might connect or interact with a particular target, such as a protein related to a disease. This helps scientists in a process called virtual screening. Instead of testing every single molecule in a lab, which would take a lot of time and resources, ML narrows down the options. It quickly points out the molecules that are most likely to be a good match. This way, scientists can focus their experiments on the most promising candidates, making drug discovery faster and more efficient.
Biomarker Discovery: Biomarkers are like signals in our body that show if we have a disease or how we respond to treatment. Biomarkers are super important because they help doctors choose the right treatment for each person. ML looks at a lot of information to find these signals. It’s like having a detective (ML) looking at all the clues (data) to figure out what makes each person unique. These clues help doctors create personalized treatments that work best for each patient.
In a nutshell, machine learning helps scientists find important signals in our body (biomarkers) so that doctors can give us the right treatment, kind of like a tailor making a custom-fit suit for each person.
Data Integration: Machine learning (ML) algorithms are like smart tools that can put together different kinds of information, such as details about genes, proteins, and information from clinical studies. The goal is to get a complete and well-rounded picture of what’s happening in a person’s body when they have a particular disease or condition.
Imagine if we only looked at one type of information, like just studying genes or only looking at clinical data. We might miss important connections or patterns.ML helps by combining all these pieces of information. It’s like putting together a puzzle where each piece is a different type of data. By doing this, scientists can discover new links and relationships that they might not see if they only focused on one thing at a time.
Deep Learning for Image Analysis: Deep learning is a type of smart computer program that’s good at understanding pictures and visual information. Deep learning is good pictures. Deep learning is like a super-smart assistant that’s excellent at understanding complicated images.
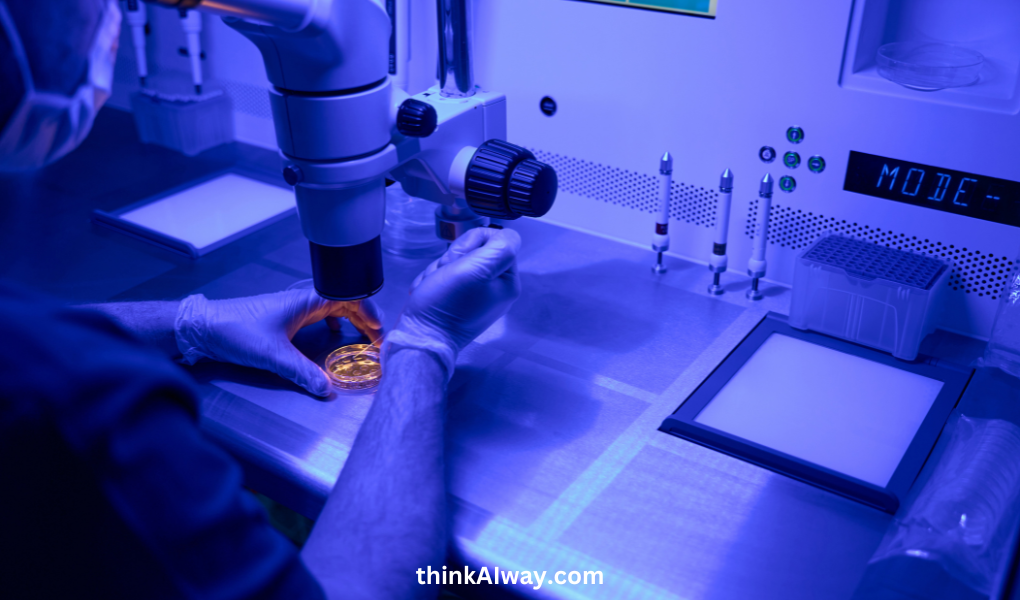
In drug discovery, scientists use deep learning to look at pictures of cells or tissues. It helps them find patterns in these images that can show if there’s something related to a disease or how a drug might work.
Patient Stratification: Machine Learning (ML) looks at various factors that make each person different, like their genes or how their body reacts to treatments.ML uses this information to group patients who are similar to each other. This helps researchers design clinical trials in a way that is more likely to work for certain groups of people.
ML helps create more personalized treatments, considering the unique features of each patient. By grouping similar patients, clinical trials become more focused. This increases the chances of finding successful treatments for specific groups of people.
In a nutshell, machine learning is like a guide that helps doctors and researchers understand what makes each person different. This way, they can create treatments and conduct clinical trials that are more likely to work for specific types of people.
Future trends of AI in drug discovery
In the future, the most significant change, as mentioned by Friedrich Rippmann, is to make the process of finding and developing new medicines faster and with fewer failures. This means more new and effective drugs can reach patients quickly.
According to experts, Using artificial intelligence (AI) in drug discovery has a lot of potential. However, right now, the high costs sometimes make it difficult to take full advantage of these opportunities. The good news is that as more companies start competing in this area, the costs are expected to go down. This means we can look forward to drug discovering new and exciting things in various fields, thanks to more affordable AI solutions.